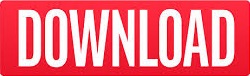
For the genes this means to identify the non-coding genomic DNA sequences that participate in the control of gene expression, and for the regulators this means to identify which protein-coding genes encode TFs, RNA binding proteins, and other regulators, as well as to determine the complete collection of regulatory RNA molecules. In order to map and characterize gene regulatory networks, one needs to first identify the nodes. Gene regulatory networks are directional because regulators control genes and usually not the other way around. They are bipartite because there are two types of nodes: genes and regulators, although of course some genes are themselves regulators of other genes or proteins.
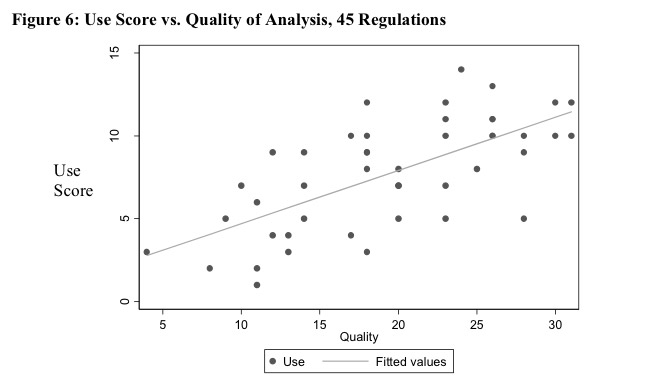
Gene regulatory networks are different from better-known protein–protein interaction networks, because gene regulatory networks are both bipartite and directional.

The edges are the physical and/or regulatory relationships between the nodes ( Fig. The network nodes are the players involved, that is, the genes and their regulators. Gene regulatory networks are composed of two main components: nodes and edges. Walhout, in Methods in Cell Biology, 2011 II Gene Regulatory Networks Current GRN theory devolves from multiple earlier roots which we very briefly trace.Īlbertha J.M. This chapter further includes a first principles quantitative treatment of network dynamics, which rationalizes the measurable kinetics of accumulation of transcriptional products and permits computational assessment of the outputs of regulatory gene cascades. The genomic regulatory transactions linked together in GRNs are executed by cis-regulatory modules, and their combinatorial information processing function deeply affect GRN organization. We consider two major aspects of GRN output, the generation of regulatory states that in turn determine all downstream genetic functions, and the Boolean nature of spatial gene expression that underlies developmental process. Here we provide an introductory overview, specifying the components of GRNs, and focusing on higher level design features such as hierarchy, modular organization, and the unidirectionality of these encoded regulatory systems. Gene regulatory network (GRN) theory defines the principal structural and functional properties of genomic control programs in animals. Peter, in Genomic Control Process, 2015 Abstract
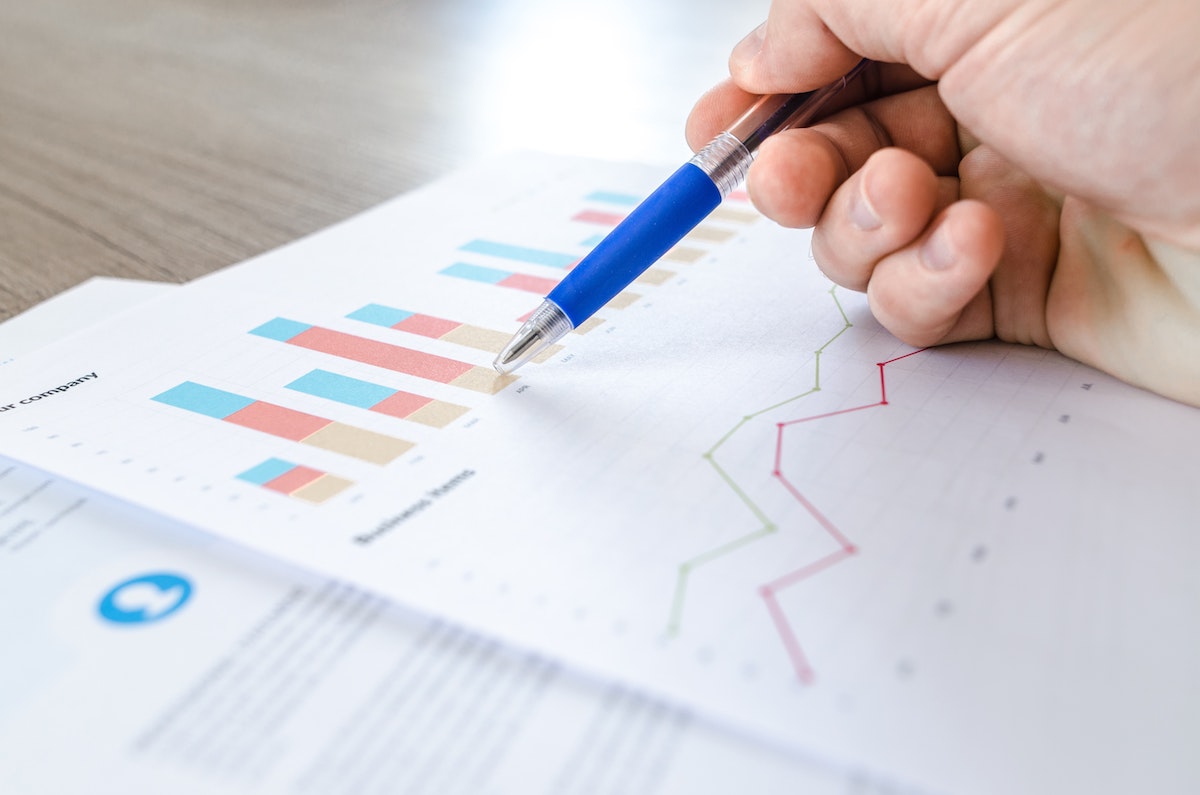
Finally, we looked ahead to the challenge of GRN construction in multiomic integration and application trends of GRNs in a clinical context.Įric H.

The consistency assessment and the validation of GRN-inferred are illustrated. Advantages, drawbacks, and application of each method were then analyzed and discussed. In this paper, we generalized the gene-regulated patterns first, and then reviewed the topology inference models/methods of GRNs from high-throughput omics data. A good GRN inference algorithm can identify correct regulatory relationships among genes, which would hugely facilitate unveiling the fundamentals of how a cell operates and functions, thus allowing novel and better understanding of diseases initiation and progression. Recent advances in high-throughput biological data collection have provided novel platforms for understanding of GRNs, thus creating an enormous interest in mathematically modeling of biological networks. Gene regulatory networks (GRNs) play a key role in various cellular processes and pathways. Lijun Cheng, in Encyclopedia of Bioinformatics and Computational Biology, 2019 Abstract
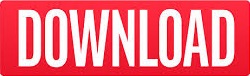